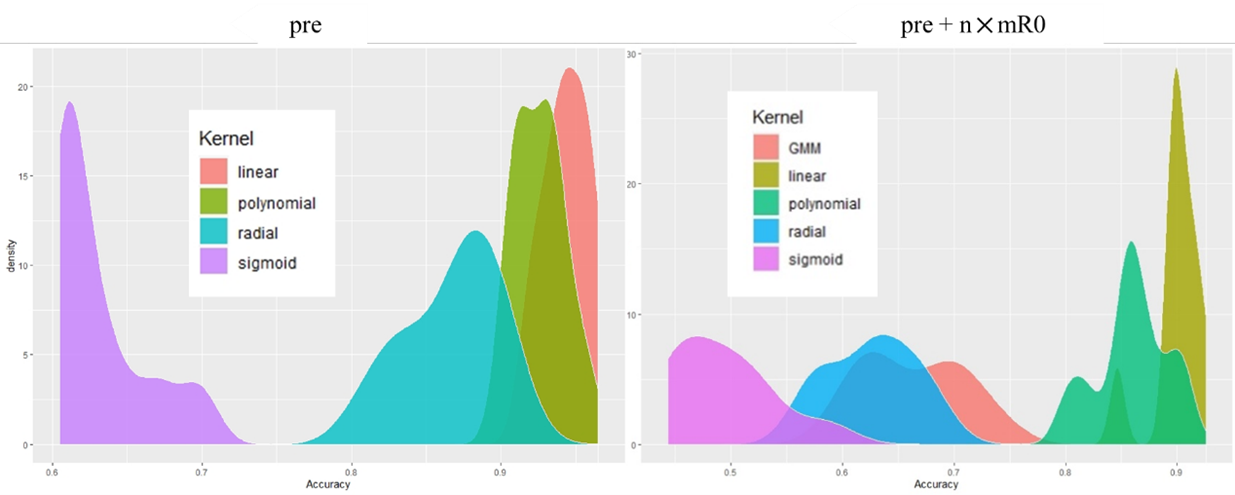
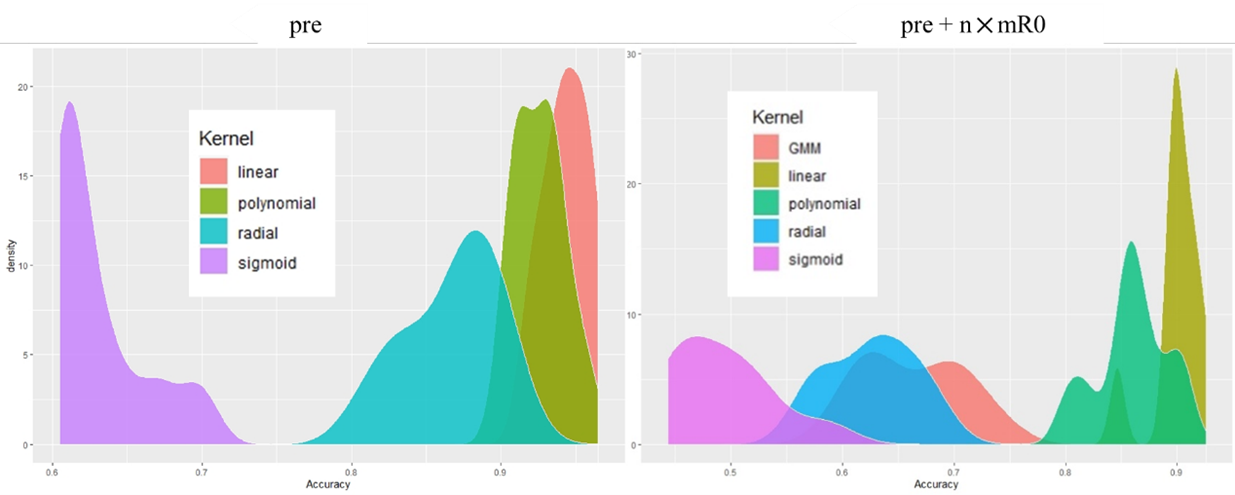
Due to the excessive number of features, a 10-fold cross-validated SVM-RFE was used to rank the importance of the features after extracting them, and then the features were added sequentially for LDA classification to record the change in accurancy with the number of features selected, and finally the best number of features was recorded as the input for the subsequent classifications (see Fig. 8). The highest accurancy for LDA classification was 89.2% (pre) / 95.6% (pre + n×mR0).
Since none of the MFCCs extracted with a fixed number of windows achieved better results than the GMM fitting method for LDA classification (6-window: 86.6%; 10-window: 88.5%; 100-window: <80%), we tested the effectiveness of the other classifiers using only the features extracted by the GMM fitting method. In this test, we randomly selected 20% of the data as the test set, and the rest of the data were used to train the classifier, which were repeated 10 times for each kernel function to record the distribution of the accuracy. Among them, the classification effect of GMM is poor when using only pre as MRU, while the effect is generally better than using only pre when using pre + n×mR0 as MRU.
There are many classifiers that ca be used for individual recognition. Considering the performances and possibilities of the classifiers, this research compared the classification effectiveness of three classifiers that have been developed considerably in the field of gibbon bioacoustics or human sound pattern recognition, i.e., (1) linear discriminant analysis (LDA), (2) support vector machine (SVM) and (3) GMM (classification by determining the similarity between the data to be measured and the existing data).
The basic method of sound pattern characteristics extraction has been identified, and a preliminary system method for individual sound recognition of Hainan gibbons has been established. Our preliminary results show that the existing system method is relatively reliable, and is to achieve the expected goals of the project. Among them, using pre + n×mR0 as MRU, extracting sound pattern characteristics using GMM fitting method, and using linear SVM for classification would be more effective. In the follow-up work, the data of rare individuals will be constantly supplemented, and design of the algorithm system will be improved, the ability of the classifier to recognise unknown individuals will be given, and the performance of the system will be comprehensively evaluated, so as to ultimately realise the recognition of individual sound of Hainan gibbons.